Inria - Institut national de recherche en sciences et technologies du numérique
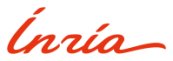
Nos dernières publications
Une minute avec François Clautiaux
CLAUTIAUX François
Rencontre avec François Clautiaux, responsable de l'équipe-projet Edge du Centre Inria de l'université de Bordeaux.
Webinaire « Retour d’expérience » de scientifiques « chiche » d’aller dans les classes – RDV du 17 …
GUILLEM Eloïse
TALBOT Hugo
VIVIAN Robin
Webinaire « Retour d’expérience » de scientifiques « chiche » d’aller dans les classes – RDV du 17 juin 2025
Webinaire « Retour d’expérience » de scientifiques « chiche » d’aller dans les classes – RDV du 17 …
FORTUN Solenn
HéLOUëT Loïc
PADOIS Vincent
Webinaire « Retour d’expérience » de scientifiques « chiche » d’aller dans les classes – RDV du 17 octobre 2024.
Une minute avec Alice Pellet-Mary
PELLET--MARY Alice
Rencontre avec Alice Pellet-Mary, chargée de recherche au sein de l'équipe-projet Canari du Centre Inria de l'université de Bordeaux.
Une minute avec Julien Dupeyrat
DUPEYRAT Julien
Rencontre avec Julien Dupeyrat, acheteur public au sein du Centre Inria de l’université de Bordeaux.
Egalité femme / homme dans l'Enseignement Supérieur et la Recherche : a-t-on vraiment évolué ?
VAUGLIN Isabelle
Isabelle Vauglin, Astrophysicienne, est Vice-Présidente de l'association Femmes et Sciences.
Pas de panique, je vous explique ! Sécuriser les méthodes réflexives
THOMAS Iona
Dans un langage de programmation, les opérations réflexives sont des outils très puissants. Elles permettent par exemple de modifier un programme en cours d’exécution, voire de modifier le langage lui
Désassemblons le numérique - #Episode13 : Déchets, alimentation, carbone - Explorer notre impact g…
BRASIER Eugénie
FERRON Aymeric
ASSOR Ambre
Dans ce nouvel épisode de Désassemblons le numérique, réalisé à l'occasion de la Journée mondiale zéro Déchet, nous allons explorer comment la recherche en visualisation immersive peut nous aider à
Une minute avec Coralie Muller
MULLER Coralie
Rencontre avec Coralie Muller, doctorante au sein de l’équipe-projet Pléiade du Centre Inria de l’université de Bordeaux.
Angela Dai – 3D in a Large-Data World
DAI Angela
Recent advances in machine learning have shown remarkable progress in the 2D and video domain, fueled by very large-scale data and compute. 3D, however, which is critical for applications spanning
Rencontre avec Julie Everaert, artiste plasticienne
EVERAERT Julie
Julie Everaert est artiste plasticienne, et mène des recherches en arts et sciences au laboratoire d’hydrodynamique de l’école Polytechnique
Pas de panique, je vous explique ! l’optimisation du cloud
JACQUET Pierre
Sur les serveurs du cloud, les ressources de calcul et de mémoire sont sous-utilisées. Les machines virtuelles souscrites par les clients fonctionnent rarement à pleine puissance. Pourrait-on fournir
Intervenants et intervenantes
Docteur en informatique (Paris 11, 1985)
Directeur de recherches à l'Inria et à l'ENS Paris, membre du collège de l'Arcep (en 2022)
Professeur au Collège de France (2011-2012) du cours "Sciences des données : de la logique du premier ordre à la Toile" dans le cadre de la chaire annuelle "Informatique et sciences numériques"
Doctorat de mécanique ; directeur de thèse en Automatique et productique à Grenoble Alpes en 2015
enseignant chercheur au Département d'Informatique de l'Université de Reykjavik en 2011
Ph. D. (Université de Cambridge, 1977)
Mathématicien. En poste au Department of statistics, University of California, Berkeley, California, USA (en 1985)
Informaticien. Chargé de recherche HDR INRIA, équipe Compilation et analyse, logiciel et matériel (CASH), Laboratoire de l'informatique du parallélisme (LIP, UMR5668 / URA1398), École normale supérieure, Lyon (2025)
Titulaire d'un doctorat de Mathématiques et Informatique, spécialité : Informatique, Bordeaux 1 (en 2007). - Maître de conférences, Université de Lorraine, LORIA (en 2018). - Professeur en informatique, Université de Lorraine (en 2024)
Professeur à la faculté EECS (Electrical Engineering and Computer Science) de l'Université de Berkeley (2019)
Chercheur en Informatique
Docteur en Sciences (Université Paris-Dauphine, 2013)
Chercheur à l'INRIA Grenoble-Rhone-Alpes en 2020
Auteur d'une thèse en Mathématiques appliquées et calcul scientifique à Bordeaux en 2022